Hive AI: Leading the Way in AI-Powered Content Understanding and Generation
Hive AI has established itself as a trailblazer in the realm of cloud-based artificial intelligence (AI) solutions, offering cutting-edge technologies to understand, search, and generate content. Trusted by hundreds of the world’s largest and most innovative organizations, Hive AI provides developers with a suite of pre-trained AI models that serve billions of customer API requests every month. In addition to these models, Hive AI also offers turnkey software products powered by proprietary AI models and datasets, enabling groundbreaking applications in content moderation, brand protection, ad targeting, and more.
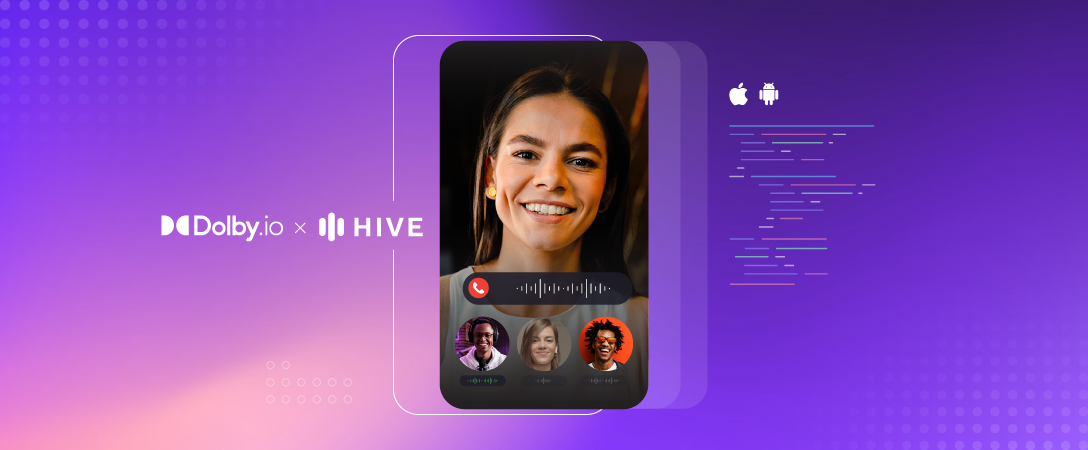
Revolutionizing Content Moderation and AI Applications
Hive AI’s technology suite is transforming traditional approaches to platform integrity, content moderation, brand protection, sponsorship measurement, and context-based ad targeting. One notable feature is its AI-generated content detection capability, which is essential in today’s digital landscape where synthetic media can be challenging to detect and moderate.
Hive’s expertise was recently showcased at the Workshop on Multimodal Content Moderation during the Computer Vision and Pattern Recognition (CVPR) conference. At this event, Hive’s Chief Technology Officer, Dmitriy, discussed several critical considerations when building machine learning models for classification tasks, including the impact of data quality and quantity, the potential use of synthetic data, and strategies to identify and mitigate bias in model performance.
The Critical Role of Quality Data in AI Development
In machine learning, data is the cornerstone of model training and performance. The general consensus in the field is that more data usually leads to better models. However, the quality of this data is equally, if not more, important. This principle applies to both machine learning models and human learning: more examples facilitate easier learning, but if the examples are flawed or noisy, the learning process becomes significantly harder.
To explore this, Hive AI conducted an experiment by training a binary image classifier to detect not-safe-for-work (NSFW) content. They varied the data size from 10 images to 100,000 images and manipulated the noise level by flipping a percentage of the labels on 0% to 50% of the data. The results were clear: the best model performance was achieved with the largest dataset (100,000 examples) and the cleanest data (0% noise). Beyond a certain point, however, adding more noisy data did not improve model accuracy, demonstrating that data quality is paramount, especially as the difficulty of the classification task increases.
This finding underscores the importance of clean data over sheer quantity. For tasks that involve complex classifications, ensuring high-quality data becomes even more crucial. This aligns with Hive AI’s strategy of leveraging high-quality datasets to train its models, thereby ensuring optimal performance in real-world applications.

Synthetic Data in AI Training: A Double-Edged Sword?
While having ample clean data is essential, what can be done when such data is scarce or expensive? With the rise of AI-generated content, many companies are now using synthetic data to supplement their training datasets. But does this synthetic data help or hinder model performance?
Hive AI investigated this by training five different binary classification models to detect smoking. Three of these models were trained exclusively on real data, while one was trained on a mix of real and synthetic data, and another entirely on synthetic data. The models were evaluated using balanced test sets consisting of real and synthetic images. The results showed that while the model trained on the largest entirely real dataset (40,000 images) performed the best, the model trained on a combination of real and synthetic data (10,000 real and 30,000 synthetic images) outperformed the one trained only on 10,000 real images.
These findings suggest that synthetic data can indeed be beneficial, particularly when real data is limited. However, the quality and realism of the synthetic data are crucial. If the synthetic data closely mimics the characteristics of real data, it can effectively enhance model performance. Hive AI’s research indicates that strategic use of synthetic data can be a valuable tool in training robust AI models.
Hive AI’s Commitment to Excellence in AI Innovation
Hive AI’s commitment to innovation in AI-driven content understanding and generation is evident in its ongoing research and development efforts. By focusing on the importance of data quality, exploring the potential of synthetic data, and addressing bias in AI models, Hive AI continues to push the boundaries of what is possible with AI technology. Their work not only enhances the capabilities of their own AI models but also sets new standards for the industry as a whole.
As AI continues to evolve, Hive AI remains at the forefront, providing powerful, reliable, and ethically sound solutions that help organizations navigate the complex landscape of digital content and communication.
